Innovative cancer researchers are using data-driven protocols to improve disease detection and treatment
Three technology innovators discussed how data-driven learning systems can be applied to different cancers to improve patient symptoms, outcomes, and overall care during the Annual Meeting symposium Electronic Health Records and Cancer Learning Health Systems.
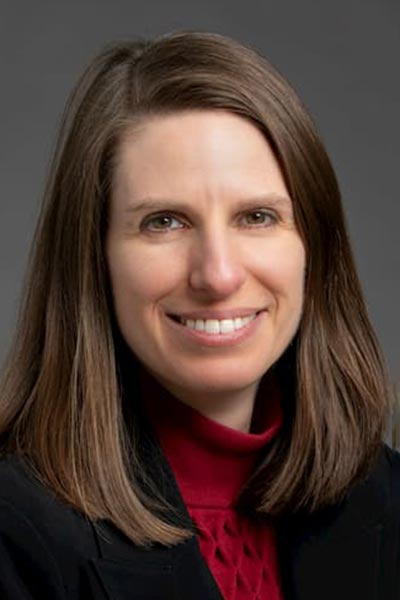
The session was presented Tuesday, April 18, and can be viewed on the virtual meeting platform by registered Annual Meeting participants through July 19, 2023.
Mia A. Levy, MD, PhD, Chief Medical Officer at Foundation Medicine, presented a learning system for breast cancer risk management. Levy and colleagues used demographic, genetic, and diagnostic data from 82,000 patients with breast cancer seen at Rush University Medical Center to create a model that could identify women who are at high risk for developing breast cancer.
The ultimate goal is to create a standardized screening protocol to ensure that high-risk individuals are notified early and made aware of recommended treatments, Levy said.
Michael Hassett, MD, MPH, Chief Quality Officer, Medical Oncologist, and Researcher at Dana-Farber Cancer Institute, developed an electronic symptom management (eSyM) tool in collaboration with the Symptom Management Implementation of Patient Reported Outcomes in Oncology (SIMPRO) trial.
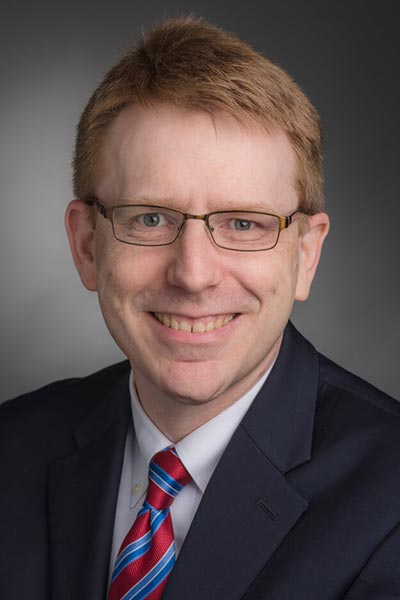
“The work that I’m talking about today really stems from the belief that a fundamental responsibility that we have as clinicians is to do as much as we can to reduce the symptom burden for cancer patients,” Hassett said.
The eSyM tool utilizes self-reported patient symptoms. After beginning treatment, patients are prompted to report their symptoms several times a week through MyChart. The results are stored in a database and patient progress is charted over time. If reported symptoms hit a severity threshold, patients are prompted to contact their physicians. Health care providers also receive alerts to check on patients and intervene early in symptom management.
Hassett cited a case study of a patient who reported severe nausea and vomiting after beginning chemotherapy. This triggered an alert for her nurse, who contacted her and administered fluids. The patient quickly stabilized and was able to continue treatment on a modified regimen.
“We’re trying to capture these … symptom events before they become serious, intervene, give supportive care, make adjustments to treatment if we can, and then move on with treatment,” Hassett said.
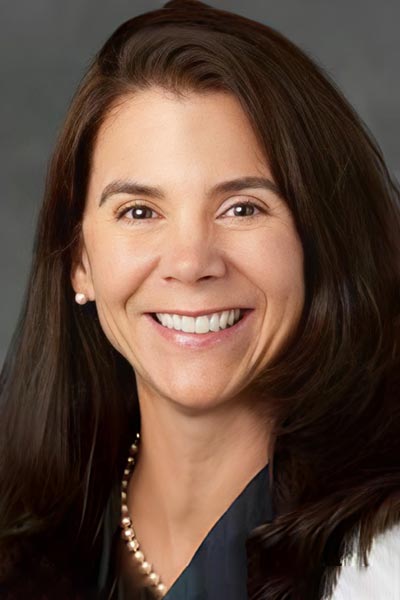
Session chair Tina Hernandez-Boussard, PhD, MPH, Associate Dean of Research and Professor of Medicine (Biomedical Informatics), Biomedical Data Sciences, and Surgery at Stanford University, described her efforts to create a predictive model for cancer symptoms using artificial intelligence (AI).
Two major challenges in cancer care are chemotherapy-induced side effects and cancer inequities, she said, and AI predictive models can help address both challenges. The AI model can give providers “a more precise way of targeting individuals who might be at higher risk for acute care utilization. That would allow us to lower costs, prevent these episodes, and really advance the quality of care for our patients,” Hernandez-Boussard said.
Thus far, the model predicts real-life outcomes to a reasonable extent, Hernandez-Boussard said, but more work is needed to implement and test the model in real-world settings and to improve its performance across different demographic groups.
Register Today for the AACR Annual Meeting 2025
Don’t miss the most important cancer meeting of the year, April 25-30 in Chicago, Illinois. In-person and virtual registration packages include full access to live sessions, Q&A, networking, CME/MOC credits, and more.
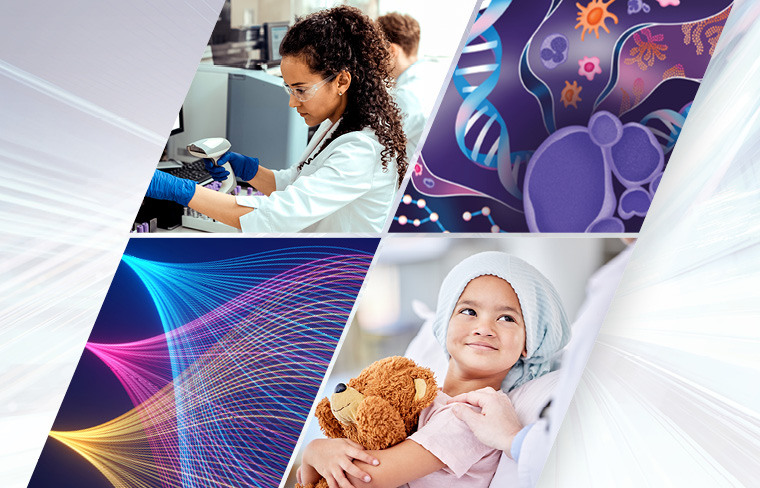