Symposium explores role of radiomics, AI analysis in cancer imaging
During an AACR 2021 symposium, Artificial Intelligence in Cancer Imaging and companion panel discussion, speakers reviewed the application of radiomics to breast, pancreatic, and lung cancers for pathodiagnosis, prognosis, and prediction of response to immune therapies. A recording of the symposium and the panel discussion will be available to registered attendees until June 21.
Radiomics and machine learning of breast cancer in diagnosis and therapeutic response
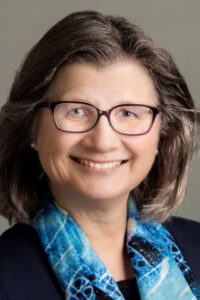
Maryellen L. Giger, PhD, University of Chicago, discussed the development, validation, and implementation of AI in the interpretation of breast cancer imaging.
“AI has been investigated for decades, with focus on both human-engineered algorithms and deep learning methods,” Giger said. “These investigations have included development of the AI algorithm, as well as the evaluation of the radiologist when being aided by the AI in order to assess translation to clinical practice.”
Various AI methods are evolving as aids to radiologists as a second reader, or a concurrent reader, or even as a primary autonomous reader; for example, as an aid in triage, she said.
“In breast cancer imaging, AI can be applied for many medical decision-making tasks—risk assessment, screening, diagnosis, prognosis, treatment planning, assessing treatment response, patient management, and disease discovery,” Giger said. “Looking at AI in the management of the breast cancer patient, specifically looking at intra-tumor heterogeneity, we can assess the tumor across different breast cancer subtypes, assess response to therapy, and risk of recurrence.”
As tasks for using AI in clinical interpretation continues to expand, Giger said it’s important to continue interdisciplinary development of the technology.
“We need to keep evaluating both the standalone performance of the AI, as well as how the end-user performs when they use AI, and we need to make sure those performances are robust in order to translate to the clinical arena,” Giger said. “We need to make sure the biomedical question is being answered by the AI and it’s being used appropriately. It’s a very promising future but more work still needs to be done.”
Quantitative imaging of pancreatic cancer from macroscopic to microscopic scales
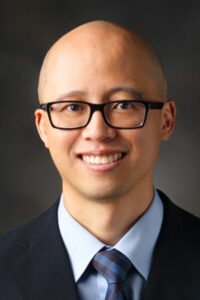
Eugene Jon Koay, MD, PhD, UT MD Anderson Cancer Center, discussed the baseline imaging and pathology of pancreatic cancer and how AI-assisted imaging analyses may lead to personalized methods for early detection.
“Currently, early detection focuses on high-risk individuals, and most of these patients undergo yearly intervals for imaging, such as with MRI,” Koay said. “What we want to do is take the oncophysics idea that cancer is a physical phenomenon of multi-scale dysregulation, as well as use mathematical models to integrate into clinical and biological systems, to help explain observations in patients who undergo screening for pancreatic cancer.”
By applying machine learning-based tools and other image segmentation analysis tools, Koay said they investigated microscopic pathological correlations of tumors and have identified quantitative imaging signals that are relevant to both pancreatic and liver cancers.
“Our approach has been to identify clinically relevant signals from the imaging for both pancreatic and liver applications,” he said. “This involves biological interrogation using the CT and MRI images and has applications toward both early detection and treatment response.”
Koay said that next steps include establishing the infrastructure for these quantitative imaging tools and working towards integrating the use of federated approaches into their image analyses.
“What we eventually hope to be able to accomplish is a more distributed or federated approach that allows us to move the imaging analysis tools or AI-based tools from a central source to other places,” he said. “In this distribution model, instead of moving the data to the model, we are now moving the model to the data.”
Radiomics of immune therapy in NSCLC
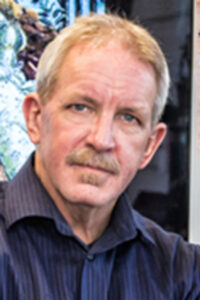
Robert J. Gillies, PhD, Moffitt Cancer Center, discussed ongoing studies involving the use of CT and PET-CT radiomics to identify PD-L1 status, which could serve as a predictive biomarker of immunotherapy response in non-small cell lung cancer (NSCLC).
In a study of PET-CT radiomics, Gillies and his colleagues curated datasets from more than 800 NSCLC patients from four international institutions. Two residual convolutional neural network models were generated to predict EGFR mutation and PD-L1 positive status in training and validation cohorts and were subsequently tested on external cohorts.
“We used a three-layer deep learning model to develop a deep learning score to predict EGFR mutation status or PD-L1 expression status,” he said. “The plan is to take these scores and use them to predict EGFR and PD-L1 status and use them in a decision support system.”
Gillies noted that the deep learning score-based prediction of PD-L1 status correlated with the score obtained using immunohistochemistry for predicting response.
Looking at EGFR mutation status, as with PD-L1 status, the deep learning score is indistinguishable from PCR-derived EGFR mutation status in predicting progression free survival, so in a way, this can substitute directly for the PCR studies or the biopsy,” Gillies said. “The combination of EGFR and PD-L1 scores generated from the same PET-CT scan can be used as a non-invasive decision support tool for NSCLC.”